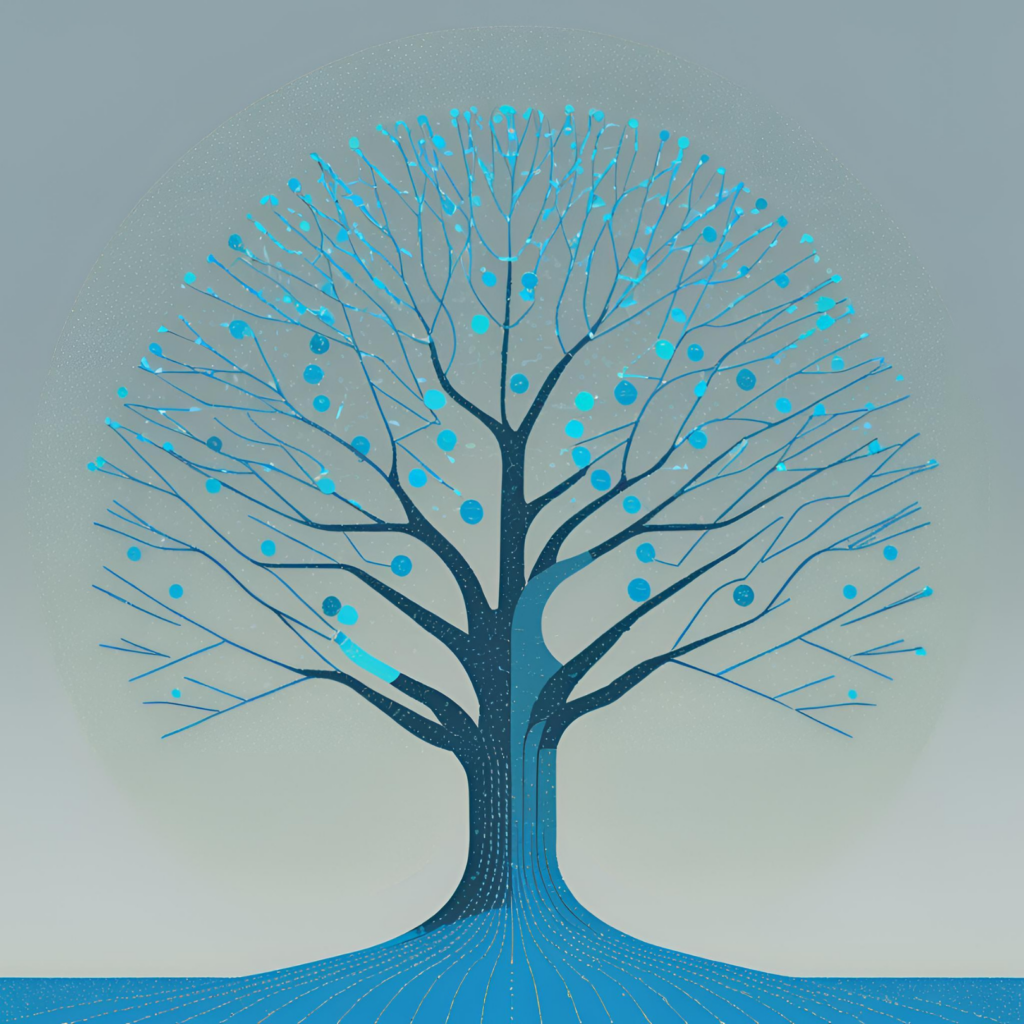
Introduction: AI’s Revolutionary Growth and Its Underlying Risks
Artificial intelligence has made rapid advancements, revolutionizing industries with powerful language models like GPT-4 and image generators such as Stable Diffusion. These models have achieved impressive results across various tasks. However, a new study highlights a critical challenge: when AI models are trained on data generated by other AI systems, they begin to lose their ability to represent the real world accurately. This gradual decline, called “model collapse,” could have far-reaching consequences for AI applications.
What Is Model Collapse? A Degenerative Learning Process
Model collapse occurs when AI models trained on AI-generated data progressively lose information about the original distribution. As training continues across multiple generations, models begin omitting rare or less common features, leading to a shrinking range of possible outputs. Over time, this results in models that fail to capture the richness and diversity of human-created data, leading to monotonous and inaccurate results.
Causes of Model Collapse: Compounding Errors in AI Training
Three main types of errors drive model collapse:
- Statistical Approximation Errors: AI learns from a finite dataset, limiting its accuracy compared to real-world distributions.
- Functional Expressivity Errors: AI models struggle to fully replicate the complexity of human language and images.
- Functional Approximation Errors: Small imperfections in the learning algorithms lead to compounded inaccuracies over generations.
These errors accumulate, resulting in AI models that gradually deviate from reality, producing misleading content.
Real-World Implications: The Danger of AI Training on AI-Generated Data
As AI-generated content becomes more prevalent online, future AI models may unknowingly train on polluted data, reinforcing mistakes and reducing creativity. This could affect search engines, misinformation detection, and automated decision-making, leading to misleading or biased outcomes. The loss of diverse perspectives in AI models is particularly concerning for marginalized communities and complex analytical tasks.
Solutions: Preventing AI Models from Degrading
To counteract model collapse, researchers suggest:
- Preserving Access to Human-Generated Data: Ensuring AI models are trained on diverse, real-world datasets helps maintain accuracy.
- Implementing Proper Data Tracking: Identifying and filtering AI-generated content from training datasets can reduce compounding errors.
- Improving AI Model Architectures: Enhancing AI learning processes can help prevent deviations from real-world complexity.
These measures will help sustain the effectiveness of AI models in the long term.
Conclusion: Ensuring Responsible AI Development
The rise of generative AI has brought impressive advancements, but its unchecked growth comes with risks. Understanding and mitigating model collapse is essential to maintaining AI’s reliability and usefulness. Organizations developing AI must invest in strategies that prioritize diverse training data and prevent AI from learning exclusively from its creations. By addressing this challenge, we can ensure that AI continues to serve society with accurate, unbiased, and meaningful insights.
Resources
Read more in AI Models Collapse When Trained on Recursively Generated Data.