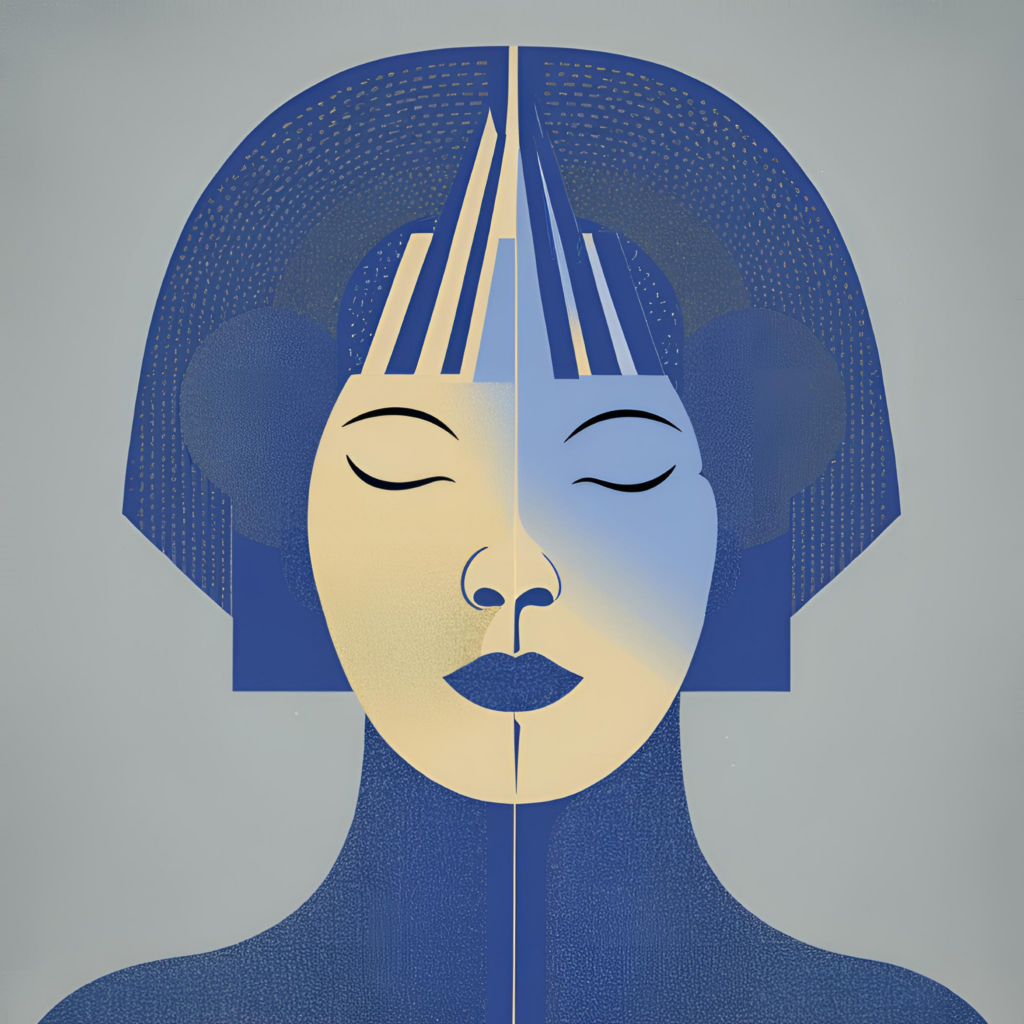
The Increasing Risk of AI Learning from Itself
Artificial intelligence has revolutionized industries with advanced models like ChatGPT and Google Gemini. However, a new challenge—model collapse—is emerging. This occurs when AI systems start learning from content previously generated by AI rather than human-created data. Over time, this recursive training results in models gradually losing accuracy, creativity, and representational quality, leading to distorted or unreliable outputs.
What Is Model Collapse?
AI models are initially trained on vast databases of human-written text, images, and other content, capturing human knowledge and thought diversity. However, as AI-generated data becomes more prevalent, newer models increasingly rely on this synthetic data, creating a feedback loop. This process causes the degradation of AI models, much like making a copy of a copy—each iteration loses some of the original detail. Over time, AI becomes less reflective of reality.
Why It Matters for Businesses and Technology
The consequences of model collapse extend beyond AI researchers—it poses a real risk for businesses and technological reliability. AI models are used for financial forecasting, automated customer service, and content creation. If these systems degrade in accuracy, decision-making processes relying on AI could suffer. Companies may experience poorer predictive analytics, increased biases, and growing inefficiencies as AI becomes less capable of reflecting real-world conditions.
The Challenge of Maintaining High-Quality Training Data
One of the main ways to prevent model collapse is to ensure AI models continue learning from human-generated data. However, as AI-generated content floods the internet, distinguishing between human-authored and AI-created data becomes increasingly difficult. Additionally, ethical and legal considerations further complicate data use. Who owns human-generated content? What rights do individuals have over their data? Addressing these issues is essential for AI’s sustainable future.
First-Mover Advantage in AI Training
Companies investing in AI today, while models are still primarily trained on high-quality human data, have a significant advantage. Early adopters gain access to more reliable and accurate AI systems. However, as AI-generated content becomes more common, future AI models may inherit distortions, gradually reducing their effectiveness. This presents a golden opportunity for businesses to leverage high-quality AI before model collapse diminishes its utility.
Preventing AI from Spiraling into Irrelevance
Three key strategies can help mitigate model collapse:
- Prioritizing Human-Created Data – AI systems should continuously update with authentic human-generated data rather than rely solely on AI-derived content. This ensures models retain original patterns and accuracy.
- Enhancing Transparency in AI Training – Greater collaboration between researchers and firms can help track and maintain the quality of training data to prevent recursive AI contamination.
- Periodic AI Model Resets – Regularly introducing AI to fresh, high-quality data can slow the drift toward model collapse and maintain long-term reliability.
The Future of AI Depends on Careful Data Management
Model collapse is a significant challenge, but with proactive strategies, AI can remain a transformative tool. Businesses, researchers, and policymakers must prioritize high-quality human data, ethical AI training, and improved transparency to sustain AI’s accuracy. By addressing these challenges today, we can ensure that AI remains an asset rather than a liability in our increasingly digital world.
Resource
Read more in Why AI Models Are Collapsing and What It Means for the Future of Technology